The unprecedented rise of artificial intelligence (AI) has brought transformative possibilities across the board, from industries and economies to societies at large. However, this technological leap also introduces a set of potential challenges. In its recent public meeting, the National AI Advisory Committee (NAIAC)1, which provides recommendations around the U.S. AI competitiveness, the science around AI, and the AI workforce to the President and the National AI Initiative Office, has voted on a recommendation on ‘Generative AI Away from the Frontier.’2
This recommendation aims to outline the risks and proposed recommendations for how to assess and manage off-frontier AI models – typically referring to open source models. In summary, the recommendation from the NAIAC provides a roadmap for responsibly navigating the complexities of generative AI. This blog post aims to shed light on this recommendation and delineate how DataRobot customers can proactively leverage the platform to align their AI adaption with this recommendation.
Frontier vs Off-Frontier Models
In the recommendation, the distinction between frontier and off-frontier models of generative AI is based on their accessibility and level of advancement. Frontier models represent the latest and most advanced developments in AI technology. These are complex, high-capability systems typically developed and accessed by leading tech companies, research institutions, or specialized AI labs (such as current state-of-the-art models like GPT-4 and Google Gemini). Due to their complexity and cutting-edge nature, frontier models typically have constrained access – they are not widely available or accessible to the general public.
On the other hand, off-frontier models typically have unconstrained access – they are more widely available and accessible AI systems, often available as open source. They might not achieve the most advanced AI capabilities but are significant due to their broader usage. These models include both proprietary systems and open source AI systems and are used by a wider range of stakeholders, including smaller companies, individual developers, and educational institutions.
This distinction is important for understanding the different levels of risks, governance needs, and regulatory approaches required for various AI systems. While frontier models may need specialized oversight due to their advanced nature, off-frontier models pose a different set of challenges and risks because of their widespread use and accessibility.
What the NAIAC Recommendation Covers
The recommendation on ‘Generative AI Away from the Frontier,’ issued by NAIAC in October 2023, focuses on the governance and risk assessment of generative AI systems. The document provides two key recommendations for the assessment of risks associated with generative AI systems:
For Proprietary Off-Frontier Models: It advises the Biden-Harris administration to encourage companies to extend voluntary commitments3 to include risk-based assessments of off-frontier generative AI systems. This includes independent testing, risk identification, and information sharing about potential risks. This recommendation is particularly aimed at emphasizing the importance of understanding and sharing the information on risks associated with off-frontier models.
For Open Source Off-Frontier Models: For generative AI systems with unconstrained access, such as open-source systems, the National Institute of Standards and Technology (NIST) is charged to collaborate with a diverse range of stakeholders to define appropriate frameworks to mitigate AI risks. This group includes academia, civil society, advocacy organizations, and the industry (where legal and technical feasibility allows). The goal is to develop testing and analysis environments, measurement systems, and tools for testing these AI systems. This collaboration aims to establish appropriate methodologies for identifying critical potential risks associated with these more openly accessible systems.
NAIAC underlines the need to understand the risks posed by widely available, off-frontier generative AI systems, which include both proprietary and open-source systems. These risks range from the acquisition of harmful information to privacy breaches and the generation of harmful content. The recommendation acknowledges the unique challenges in assessing risks in open-source AI systems due to the lack of a fixed target for assessment and limitations on who can test and evaluate the system.
Moreover, it highlights that investigations into these risks require a multi-disciplinary approach, incorporating insights from social sciences, behavioral sciences, and ethics, to support decisions about regulation or governance. While recognizing the challenges, the document also notes the benefits of open-source systems in democratizing access, spurring innovation, and enhancing creative expression.
For proprietary AI systems, the recommendation points out that while companies may understand the risks, this information is often not shared with external stakeholders, including policymakers. This calls for more transparency in the field.
Regulation of Generative AI Models
Recently, discussion on the catastrophic risks of AI has dominated the conversations on AI risk, especially with regards to generative AI. This has led to calls to regulate AI in an attempt to promote responsible development and deployment of AI tools. It is worth exploring the regulatory option with regards to generative AI. There are two main areas where policy makers can regulate AI: regulation at model level and regulation at use case level.
In predictive AI, generally, the two levels significantly overlap as narrow AI is built for a specific use case and cannot be generalized to many other use cases. For example, a model that was developed to identify patients with high likelihood of readmission, can only be used for this particular use case and will require input information similar to what it was trained on. However, a single large language model (LLM), a form of generative AI models, can be used in multiple ways to summarize patient charts, generate potential treatment plans, and improve the communication between the physicians and patients.
As highlighted in the examples above, unlike predictive AI, the same LLM can be used in a variety of use cases. This distinction is particularly important when considering AI regulation.
Penalizing AI models at the development level, especially for generative AI models, could hinder innovation and limit the beneficial capabilities of the technology. Nonetheless, it is paramount that the builders of generative AI models, both frontier and off-frontier, adhere to responsible AI development guidelines.
Instead, the focus should be on the harms of such technology at the use case level, especially at governing the use more effectively. DataRobot can simplify governance by providing capabilities that enable users to evaluate their AI use cases for risks associated with bias and discrimination, toxicity and harm, performance, and cost. These features and tools can help organizations ensure that AI systems are used responsibly and aligned with their existing risk management processes without stifling innovation.
Governance and Risks of Open vs Closed Source Models
Another area that was mentioned in the recommendation and later included in the recently signed executive order signed by President Biden4, is lack of transparency in the model development process. In the closed-source systems, the developing organization may investigate and evaluate the risks associated with the developed generative AI models. However, information on potential risks, findings around outcome of red teaming, and evaluations done internally has not generally been shared publicly.
On the other hand, open-source models are inherently more transparent due to their openly available design, facilitating the easier identification and correction of potential concerns pre-deployment. But extensive research on potential risks and evaluation of these models has not been conducted.
The distinct and differing characteristics of these systems imply that the governance approaches for open-source models should differ from those applied to closed-source models.
Avoid Reinventing Trust Across Organizations
Given the challenges of adapting AI, there’s a clear need for standardizing the governance process in AI to prevent every organization from having to reinvent these measures. Various organizations including DataRobot have come up with their framework for Trustworthy AI5. The government can help lead the collaborative effort between the private sector, academia, and civil society to develop standardized approaches to address the concerns and provide robust evaluation processes to ensure development and deployment of trustworthy AI systems. The recent executive order on the safe, secure, and trustworthy development and use of AI directs NIST to lead this joint collaborative effort to develop guidelines and evaluation measures to understand and test generative AI models. The White House AI Bill of Rights and the NIST AI Risk Management Framework (RMF) can serve as foundational principles and frameworks for responsible development and deployment of AI. Capabilities of the DataRobot AI Platform, aligned with the NIST AI RMF, can assist organizations in adopting standardized trust and governance practices. Organizations can leverage these DataRobot tools for more efficient and standardized compliance and risk management for generative and predictive AI.
1 National AI Advisory Committee – AI.gov
2 RECOMMENDATIONS: Generative AI Away from the Frontier
4 https://www.datarobot.com/trusted-ai-101/
About the author
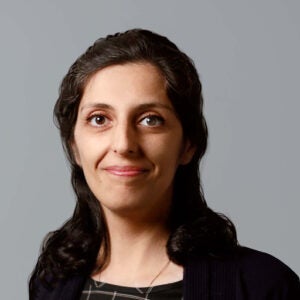
Haniyeh is a Global AI Ethicist at the DataRobot Trusted AI team and a member of the National AI Advisory Committee (NAIAC). Her research focuses on bias, privacy, robustness and stability, and ethics in AI and Machine Learning. She has a demonstrated history of implementing ML and AI in a variety of industries and initiated the incorporation of bias and fairness feature into DataRobot product. She is a thought leader in the area of AI bias and ethical AI. Haniyeh holds a PhD in Astronomy and Astrophysics from the Rheinische Friedrich-Wilhelms-Universität Bonn.
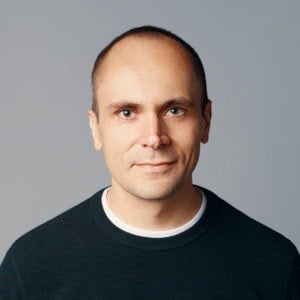
Michael Schmidt serves as Chief Technology Officer of DataRobot, where he is responsible for pioneering the next frontier of the company’s cutting-edge technology. Schmidt joined DataRobot in 2017 following the company’s acquisition of Nutonian, a machine learning company he founded and led, and has been instrumental to successful product launches, including Automated Time Series. Schmidt earned his PhD from Cornell University, where his research focused on automated machine learning, artificial intelligence, and applied math. He lives in Washington, DC.